Machine Learning in Healthcare - TOEFL iBT® Reading Practice Test
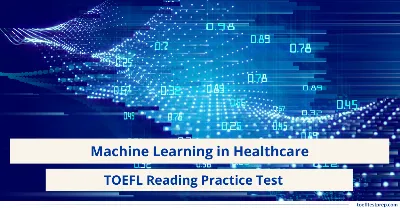
"Refine your TOEFL Reading Comprehension with our practice passage on 'Machine Learning in Healthcare,' examining AI's transformative impact on medicine."
Key Highlights
The TOEFL Reading section often covers innovative topics like 'Machine Learning in Healthcare,' highlighting the use of AI to revolutionise diagnostics, treatments, and patient care. Practising with such passages will help you understand complex ideas, analyse data-driven advancements, and improve your ability to answer comprehension questions accurately.
Reading Instructions:
- You have 15 minutes to read the following passage and answer all 9 questions related to it.
- Most questions are worth 1 point, but the last question is worth more. The directions indicate how many points you will receive for that specific question.
- The specific section/paragraphs have been provided again with the question for ease of understanding and quick solution.
- Some questions include a word or phrase that is highlighted in the question as well as in the paragraph for quick reference.
Machine Learning in Healthcare
Machine learning, a key part of artificial intelligence, has become a game changer in the
healthcare field. By using large amounts of data, machine learning methods can spot complex patterns and make predictions that traditional methods couldnt achieve. Bringing machine learning into healthcare can improve diagnosis, customize treatment plans, and make administrative tasks easier. Healthcare systems around the world are dealing with more patients and complicated medical data. Adopting machine learning technologies offers a hopeful way to enhance patient outcomes and efficiency. But using these technologies requires a good understanding of what they can and cant do.
One of the biggest uses of machine learning in healthcare is in diagnostics. For example,methods trained on large sets of medical images can help radiologists find issues like tumors or fractures with great accuracy. Research shows that machine learning models can sometimes do better than human experts in certain diagnostic areas, particularly in radiology and pathology. These models look at thousands of images, learning to tell the difference between normal and abnormal findings, which helps reduce misdiagnosis. Yet, whiletheres a lot of potential for better diagnostic abilities, there are worries about how understandable these algorithms are. Clinicians need to know how machine learning systems make decisions to trust and use them effectively, as a lack of clarity could slow down their acceptance.
Another important area where machine learning is progressing is in personalized medicine. By looking at genetic information, lifestyle factors, and how patients respond to treatments, machine learning methods can help create therapies tailored to individual patients. For instance, in cancer treatment, forecasting models can evaluate how a patient might react to specific chemotherapy based on their genetic makeup. This method not only makes treatments more effective but also reduces side effects by steering clear of therapies that wont work. However, using personalized medicine through machine learning brings up ethical issues, especially around data privacy and possible bias in predictions. Its crucial to ensure these models are trained on diverse datasets to prevent differences in healthcare outcomes, as biased algorithms could worsen existing inequalities.
Machine learning also plays a key role in forecasting analytics, which can greatly improve patient care. By examining past patient data, machine learning models can predict potential health risks, allowing for preventive measures. For example, algorithms can estimate the chance of hospital readmissions, helping healthcare providers create strategies to lower those rates. Also, machine learning can help identify patients at risk for chronic conditions like diabetes or heart disease, enabling early intervention and lifestyle changes. This way, healthcare providers can use resources more wisely, ultimately leading to better patient outcomes. However, the success of these forecasting models depends on the quality and completeness of the data used for training. Inaccurate or missing data can result in wrong predictions, which could endanger patient safety and damage trust in these technologies.
Despite the many benefits of machine learning in healthcare, there are still obstacles to its widespread use. One major obstacle is getting machine learning systems to fit with current healthcare setups. Many healthcare providers still use older systems that may not work well with advanced machine learning technologies. Plus, training healthcare professionals to use these tools effectively is essential. Without proper training, clinicians might hesitate to rely on machine learning systems, worrying they could weaken their expertise. The rules around using machine learning in healthcare are still being developed, creating uncertainty for both
developers and healthcare providers. Setting clear guidelines and standards will be important to build trust and help integrate these technologies, ensuring they are used safely and effectively.
In summary, machine learning has great potential to change healthcare by improving diagnosis, personalizing treatment, and enhancing forecasting analytics. However, the successful application of these technologies requires careful thought about ethical, regulatory, and practical challenges. As the field keeps evolving, ongoing research and teamwork among tech experts, healthcare providers, and policymakers will be crucial to making the most of machine learning. The future of healthcare may depend on how well we can integrate these advanced technologies while keeping patient safety and fairness at the forefront of medical practice. Also, as questions about the long-term effects of machine learning in healthcare remain, continued exploration and discussion will be essential to manage this fast-changing landscape.
Directions: Once you have read the passage, answer the following questions.
Paragraph 1
Machine learning, a key part of artificial intelligence, has become a game changer in the healthcare field. By using large amounts of data, machine learning methods can spot complex patterns and make predictions that traditional methods couldn't achieve. Bringing machine learning into healthcare can improve diagnosis, customise treatment plans, and make administrative tasks easier. Healthcare systems around the world are dealing with more patients and complicated medical data. Adopting machine learning technologies offers a hopeful way to enhance patient outcomes and efficiency. But using these technologies requires a good understanding of what they can and can't do.
Factual Information Questions
- According to paragraph 1, what are some benefits of incorporating machine learning into
healthcare?- It can reduce the number of patients.
- It can improve diagnosis and customise treatment plans.
- It can eliminate the need for healthcare professionals
- It can simplify medical data collection.
Negative Factual Information Questions
- The author mentions all of the following as challenges in adopting machine learning in
healthcare EXCEPT:
- The complexity of medical data.
- The need for a good understanding of the technology.
- The potential for machine learning to replace human expertise.
- The potential for machine learning to replace human expertise.
Paragraph 2
One of the biggest uses of machine learning in healthcare is in diagnostics. For example, methods trained on large sets of medical images can help radiologists find issues like tumours or fractures with great accuracy. Research shows that machine learning models can sometimes do better than human experts in certain diagnostic areas, particularly in radiology and pathology. These models look at thousands of images, learning to tell the difference between normal and abnormal findings, which helps reduce misdiagnosis. Yet, while theres a lot of potential for better diagnostic abilities, there are worries about how understandable these algorithms are. Clinicians need to know how machine learning systems make decisions to trust and use them effectively, as a lack of clarity could slow down their acceptance.
Inference Questions
- What can be inferred from paragraph 2 about the relationship between machine learning
and human experts in diagnostics?
- Machine learning will completely replace human experts.
- Machine learning can complement human expertise by improving accuracy.
- Human experts are always more accurate than machine learning models.
- Machine learning is less reliable than traditional diagnostic methods.
Rhetorical Purpose Questions
- Why does the author mention the need for clinicians to understand how machine learning
systems make decisions in paragraph 2?- To highlight the importance of transparency for effective use.
- To suggest that machine learning is too complex for clinicians.
- To argue that human intuition is more important than algorithms.
- To indicate that machine learning systems are infallible.
Paragraph 3
Another important area where machine learning is progressing is in personalised medicine. By looking at genetic information, lifestyle factors, and how patients respond to treatments, machine learning methods can help create therapies tailored to individual patients. For instance, in cancer treatment, forecasting models can evaluate how a patient might react to specific chemotherapy based on their genetic makeup. This method not only makes treatments more effective but also reduces side effects by steering clear of therapies that wont work. However, using personalised medicine through machine learning brings up ethical issues, especially around data privacy and possible bias in predictions. Its crucial to ensure these models are trained on diverse datasets to prevent differences in healthcare outcomes, as biased algorithms could worsen existing inequalities.
Vocabulary Questions
- In paragraph 3, the word tailored is closest in meaning to:
- Generalised
- Customised
- Simplified
- Standardised
Sentence Simplification Questions
- Which of the sentences below best expresses the essential information in the following
text in paragraph 3? By looking at genetic information, lifestyle factors, and how patients respond to treatments, machine learning methods can help create therapies tailored to individual patients.
- Machine learning can create therapies based on genetic and lifestyle factors
- Therapies are created without considering patient responses.
- Machine learning ignores genetic information in treatment plans.
- Individual patients do not benefit from machine learning.
Paragraph 4
Machine learning also plays a key role in forecasting analytics, which can greatly improve patient care. By examining past patient data, machine learning models can predict potential health risks, allowing for preventive measures. For example, algorithms can estimate the chance of hospital readmissions, helping healthcare providers create strategies to lower those rates. Also, machine learning can help identify patients at risk for chronic conditions like diabetes or heart disease, enabling early intervention and lifestyle changes. This way, healthcare providers can use resources more wisely, ultimately leading to better patient outcomes. However, the success of these forecasting models depends on the quality and completeness of the data used for training. Inaccurate or missing data can result in wrong predictions, which could endanger patient safety and damage trust in these technologies.
Purpose of the Passage Questions
- What is the main purpose of paragraph 4?
- To discuss the limitations of machine learning in healthcare.
- To explain how machine learning can enhance patient care through forecasting.
- To argue against the use of machine learning in predicting health risks.
- To highlight the importance of human judgment in patient care.
Paragraph 5
Despite the many benefits of machine learning in healthcare, there are still obstacles to its widespread use. One major obstacle is getting machine learning systems to fit with current healthcare setups. Many healthcare providers still use older systems that may not work well with advanced machine learning technologies. Plus, training healthcare professionals to use these tools effectively is essential. Without proper training, clinicians might hesitate to rely on machine learning systems, worrying they could weaken their expertise. The rules around using machine learning in healthcare are still being developed, creating uncertainty for both developers and healthcare providers. Setting clear guidelines and standards will be important to build trust and help integrate these technologies, ensuring they are used safely and effectively.
Insert Missing Sentence Question
- In paragraph 5, there is a missing sentence. The paragraph is repeated below and shows
four letters (A, B, C, and D) that indicate where the following sentence could be added.Additionally, the integration of machine learning requires significant investment in technology.
- Despite the many benefits of machine learning in healthcare, there are still obstacles to its widespread use.
- One major obstacle is getting machine learning systems to fit with current healthcare setups.
- Many healthcare providers still use older systems that may not work well with advanced machine learning technologies
- Plus, training healthcare professionals to use these tools effectively is essential.
Paragraph 6
In summary, machine learning has great potential to change healthcare by improving diagnosis, personalising treatment, and enhancing forecasting analytics. However, the successful application of these technologies requires careful thought about ethical, regulatory, and practical challenges. As the field keeps evolving, ongoing research and teamwork among tech experts, healthcare providers, and policymakers will be crucial to making the most of machine learning. The future of healthcare may depend on how well we can integrate these advanced technologies while keeping patient safety and fairness at the forefront of medical practice. Also, as questions about the long-term effects of machine learning in healthcare remain, continued exploration and discussion will be essential to manage this fast-changing landscape.
Prose Summary Questions
- Directions: An introductory sentence for a brief summary of the passage is provided below. Complete the summary by selecting the THREE answer choices that express the most important ideas in the passage. Some sentences do not belong in the summary because they express ideas that are not presented in the passage or are minor ideas in the passage. This question is worth 2 points.
Machine learning has the potential to revolutionise healthcare by improving various aspects of patient care.
Answer Choices
- It can enhance diagnostics, personalisation of treatment, and forecasting analytics.
- The integration of machine learning is straightforward and requires minimal training.
- Ethical and regulatory challenges must be addressed for successful implementation.
- Collaboration among stakeholders is essential for the future of healthcare.
- What is one of the main concerns regarding the use of machine learning in healthcare as mentioned in the passage?
- The technology is too advanced for current healthcare systems.
- There is a risk of biased algorithms affecting healthcare outcomes.
- Machine learning will completely replace healthcare professionals.
- The cost of implementing machine learning is prohibitively high.
Answer Key with Explanation for TOEFL Reading Passage - Machine Learning in Healthcare
1. Answer: B
Explanation: The passage clearly states that machine learning can enhance diagnosis and tailor treatment plans, highlighting its potential to improve patient outcomes. Options A, C, and D do not accurately reflect the benefits mentioned in the passage.
2. Answer: C
Explanation:The passage discusses the need for understanding machine learning andthe complexity of medical data as challenges, but it does not suggest that machine learning aims to replace human expertise. Instead, it emphasizes the importance of integrating these technologies with human skills.
3. Answer: B
Explanation: The passage indicates that machine learning models can sometimes outperform human experts in diagnostics, suggesting a complementary relationship where machine learning enhances the accuracy of human diagnostics rather than replacing them.
4. Answer: A
Explanation: The author stresses that clinicians need to understand the decision- making processes of machine learning systems to trust and effectively use them. This underscores the importance of transparency in the adoption of new technologies in healthcare.
5. Answer: B
Explanation: The term tailored refers to creating something specifically suited to individual needs, which aligns closely with customised.The other options do not convey the same meaning of personalisation.
6. Answer: A
Explanation: This option succinctly captures the essence of the original sentence, focusing on how machine learning utilises genetic and lifestyle information to develop personalised therapies, while the other options misrepresent the information.
7. Answer: B
Explanation: The paragraph discusses the role of machine learning in predicting health risks and improving patient care, making it clear that its primary purpose is to highlight the benefits of forecasting analytics in healthcare.
8. Answer: B
Explanation: The missing sentence logically follows the mention of obstacles in adopting machine learning, as it adds to the discussion about the challenges of integrating new technologies into existing systems.
9. Answer: B
Explanation: These choices reflect the key themes of the passage, including the benefits of machine learning, the need to address challenges, and the importance of collaboration. Option B is incorrect as it misrepresents the complexity of integration.
10. Answer: B
Explanation: The passage discusses ethical issues, particularly concerning data privacy and bias in predictions, indicating that biased algorithms could worsen healthcare inequalities. The other options do not accurately reflect the concerns raised in the text.
Also Read:
Featured Articles
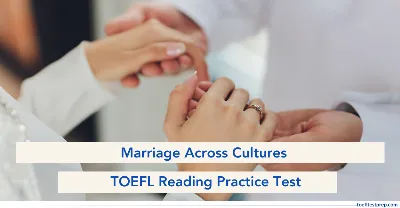
Marriage Across Cultures - TOEFL Reading Practice Test
Improve TOEFL Reading with passages on 'Marriage Across Cultures,' delving into unique traditions, values, and global perspectives on marriage.
July 15, 2025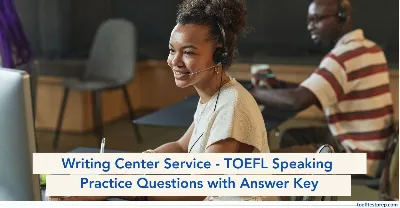
Writing Center Services - TOEFL Speaking Practice Test with Answers (Integrated)
Prepare for the TOEFL Integrated Speaking section with a practice test on 'Writing Center Services' and learn to improve score in TOEFL exam.
July 15, 2025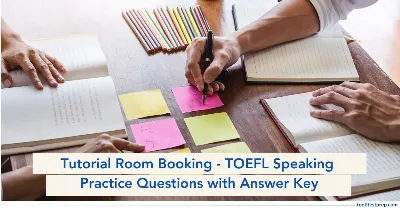
Tutorial Room Booking - TOEFL Speaking Practice Test with Answers (Integrated)
Prepare for the TOEFL Integrated Speaking section with a practice test on 'Tutorial Room Booking ' and learn to improve score in TOEFL exam.
July 15, 2025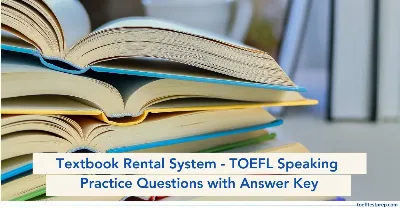
Textbook Rental System - TOEFL Speaking Practice Test with Answers (Integrated)
Prepare for the TOEFL Integrated Speaking section with a practice test on 'Textbook Rental System' and learn to improve score in TOEFL exam.
July 15, 2025